University of Texas researchers have used AI to develop a safer, effective version of an antibiotic that shows promise in animal trials. This new method could accelerate the creation of treatments for antibiotic-resistant bacterial infections. Credit: The University of Texas at Austin
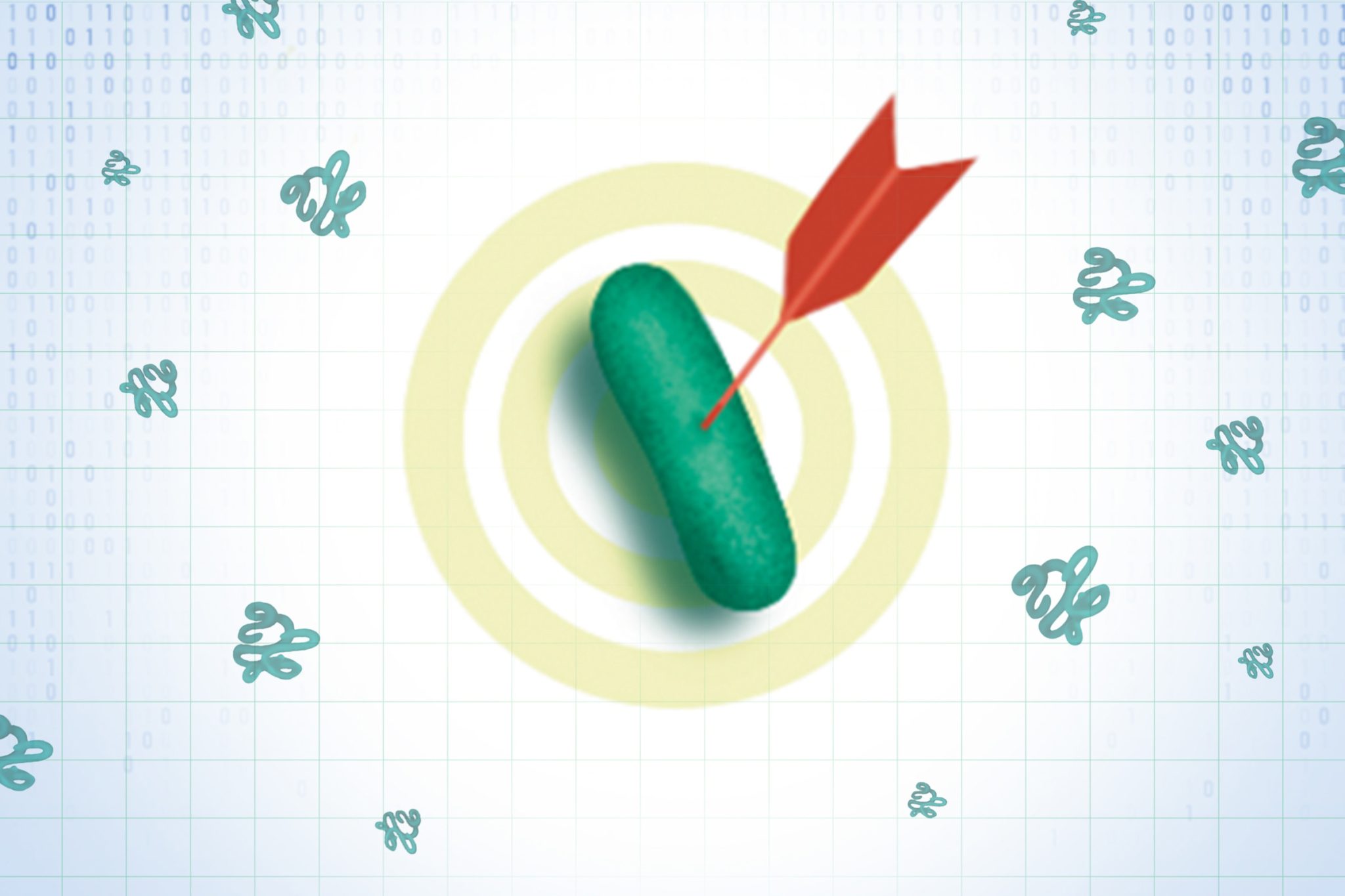
A large language model, similar to the AI that powers ChatGPT, has been utilized to develop a version of a bacteria-killing drug that was once toxic to humans.
In a hopeful development for the demand for safer and more effective antibiotics, researchers at The University of Texas at Austin have utilized artificial intelligence to create a new drug that is already demonstrating promise in animal trials.
Publishing their results in Nature Biomedical Engineering, the scientists describe using a large language model—an AI tool like the one that powers ChatGPT—to engineer a version of a bacteria-killing drug that was previously toxic in humans, so that it would be safe to use.
The prognosis for patients with dangerous bacterial infections has worsened in recent years as antibiotic-resistant bacterial strains spread and the development of new treatment options has stalled. However, UT researchers say AI tools are game-changing.
“We have found that large language models are a major step forward for machine learning applications in protein and peptide engineering,” said Claus Wilke, professor of integrative biology and statistics and data sciences, and co-senior author of the new paper. “Many use cases that weren’t feasible with prior approaches are now starting to work. I foresee that these and similar approaches are going to be used widely for developing therapeutics or drugs going forward.”
AI Applications in Drug Development
Large language models, or LLMs, were originally designed to generate and explore sequences of text, but scientists are finding creative ways to apply these models to other domains. For example, just as sentences are made up of sequences of words, proteins are made up of sequences of amino acids. LLMs cluster together words that share common attributes (such as cat, dog, and hamster) in what’s known as an “embedding space” with thousands of dimensions. Similarly, proteins with similar functions, like the ability to fight off dangerous bacteria without hurting the people who host said bacteria, may cluster together in their own version of an AI embedding space.
“The space containing all molecules is enormous,” said Davies, co-senior author of the new paper. “Machine learning allows us to find the areas of chemical space that have the properties we’re interested in, and it can do it so much more quickly and thoroughly than standard one-at-a-time lab approaches.”
For this project, the researchers employed AI to identify ways to reengineer an existing antibiotic called Protegrin-1 that is great at killing bacteria, but toxic to people. Protegrin-1, which is naturally produced by pigs to combat infections, is part of a subtype of antibiotics called antimicrobial peptides (AMPs). AMPs generally kill bacteria directly by disrupting cell membranes, but many target both bacterial and human cell membranes.
Innovating with AI for Safer Antibiotics
First, the researchers used a high-throughput method they had previously developed to create more than 7,000 variations of Protegrin-1 and quickly identify areas of the AMP that could be modified without losing its antibiotic activity.
Next, they trained a protein LLM on these results so that the model could evaluate millions of possible variations for three features: selectively targeting bacterial membranes, potently killing bacteria and not harming human red blood cells to find those that fell in the sweet spot of all three. The model then helped guide the team to a safer, more effective version of Protegrin-1, which they dubbed bacterially selective Protegrin-1.2 (bsPG-1.2).
Mice infected with multidrug-resistant bacteria and treated with bsPG-1.2 were much less likely to have detectable bacteria in their organs six hours after infection, compared to untreated mice. If further testing offers similarly positive results, the researchers hope eventually to take a version of the AI-informed antibiotic drug into human trials.
“Machine learning’s impact is twofold,” Davies said. “It’s going to point out new molecules that could have the potential to help people, and it’s going to show us how we can take those existing antibiotic molecules and make them better and focus our work to more quickly get those to clinical practice.”
Reference: “Deep mutational scanning and machine learning for the analysis of antimicrobial-peptide features driving membrane selectivity” by Justin R. Randall, Luiz C. Vieira, Claus O. Wilke and Bryan W. Davies, 31 July 2024, Nature Biomedical Engineering.
DOI: 10.1038/s41551-024-01243-1
Funding for this research was provided by the National Institutes of Health, The Welch Foundation, the Defense Threat Reduction Agency, and Tito’s Handmade Vodka.