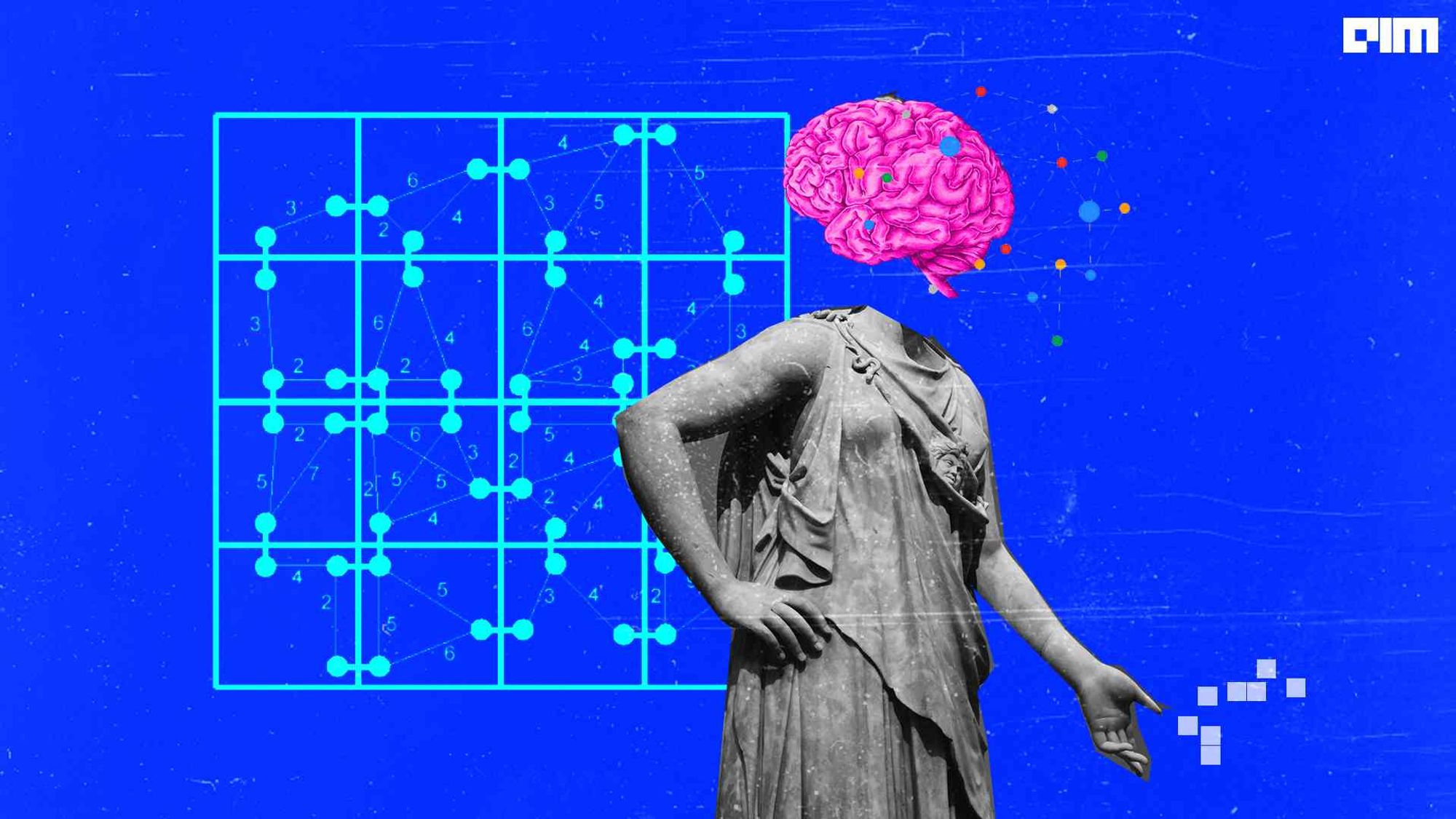
Google AI aims to apply AI to products and domains that will make AI accessible to all. To fulfil this mission, the tech giant conducts cutting-edge research to bring out innovations helpful to society. This year, too, we saw many such models and algorithms from Google.
Though not possible to focus on all, let us take a look at some of the interesting innovations that came from Google AI this year.
Wikipedia-Based Image Text (WIT) Dataset
In September, Google released the Wikipedia-Based Image Text (WIT) Dataset. It is a large multimodal dataset created by extracting multiple different text selections associated with an image from Wikipedia articles and Wikimedia image links. Google says this was followed by rigorous filtering to only retain high-quality image-text sets. The final result, as a set, has 37.5 million entity-rich image-text examples with 11.5 million unique images across 108 languages. WIT wants to create a large dataset without compromising on the quality or coverage of concepts. Google says this is the reason they moved focus to the largest online encyclopaedia available today – Wikipedia.
Image: Google
For more details, click here.
GoEmotions Dataset
The tech biggie came out with GoEmotions, a human-annotated dataset of 58,000 Reddit comments extracted from popular English-language subreddits and labelled with 27 emotion categories. These include 12 positive, 11 negative, and 4 ambiguous emotion categories and 1 “neutral” category, keeping in mind psychology and data applicability.
Google said that the GoEmotions taxonomy wants to give the greatest coverage of the emotions expressed in Reddit data and the best coverage of types of emotional expressions.
For more details, click here.
Indian Language Transliterations in Google Maps
In Google Maps, the names of most Indian places of interest (POIs) in Google Maps are not generally available in the native scripts of the languages of India. For the majority of times, they are in English or may be combined with acronyms based on the Latin script and Indian language words and names.
To solve this issue, Google came out with an ensemble of learned models to transliterate names of Latin script POIs into ten languages prominent in India. These include Hindi, Bangla, Marathi, Telugu, Tamil, Gujarati, Kannada, Malayalam, Punjabi, and Odia. Google said that with this ensemble, it has added names in these languages to millions of POIs in India, increasing the coverage nearly twenty-fold in some languages.
For more details, click here.
MetNet-2 -12-hour Precipitation Forecasting
In another achievement in the climate space, Google came out with Meteorological Neural Network 2 (MetNet-2) for 12-hour precipitation forecasting. It uses deep learning methods for forecasting by learning to predict directly from observed data.
Image: Google
It added that the computations are faster than physics-based techniques. While its predecessor, MetNet, released last year, provided eight-hour forecasting, MetNet-2 takes it up a notch higher with 12-hour precipitation forecasting.
For more details, click here.
FLAN Model
The Fine-tuned LAnguage Net (FLAN) model from Google explores a simple technique called instruction fine-tuning. This NLP model is fine-tuned on a large set of varied instructions that use a simple and intuitive description of the task. FLAN uses templates to transform existing datasets into an instructional format instead of creating a dataset of instructions from scratch to fine-tune the model.
Image: Google
For more details, click here.
Generalist Language Model (GLaM)
Google AI came out with the Generalist Language Model (GLaM), a trillion weight model that uses sparsity. The full version of GLaM has a whopping 1.2T total parameters across 64 experts per mixture of experts (MoE) layer with 32 MoE layers in total. But, it only activates a subnetwork of 97B (8% of 1.2T) parameters per token prediction during inference.
GLaM’s performance compares favourably to GPT-3 (175B), with significantly improved learning efficiency across 29 public NLP benchmarks in seven categories. This spreads over language completion, open-domain question answering, and natural language inference tasks.
Image: Google
As for the Megatron-Turing model, GLaM is on-par on the seven respective tasks if using a 5% margin while using 5x less computation during inference.
For more details, click here.