
The AIVAS supercomputer. ALAFIA AI
Healthcare applications such as digital pathology, neurology, genetics research, and cardiac imaging are becoming increasingly reliant on advanced computational tools.
To address these growing demands, Florida-based medical equipment manufacturing company ALAFIA AI has developed AIVAS, a high-performance personal supercomputer designed to accelerate AI workflows in clinical and research settings, unveiled at CES 2025.
Streamlining AI in medicine
AIVAS delivers over 1,000 trillion operations per second (TOPS), making it ideal for handling data-intensive medical tasks. From facilitating next-generation sequencing algorithms to processing complex imaging data like PET scans, the supercomputer is tailored to enhance accuracy and efficiency in critical healthcare operations and help researchers benefit from faster insights and streamlined workflows.
Camilo Buscaron, ALAFIA’s founder and a veteran of NVIDIA and AWS, explains that AIVAS was developed to bridge the gap in the infrastructure needed for AI in healthcare. He notes that as medical data generation continues to grow, robust systems like AIVAS are essential for turning that data into actionable insights.
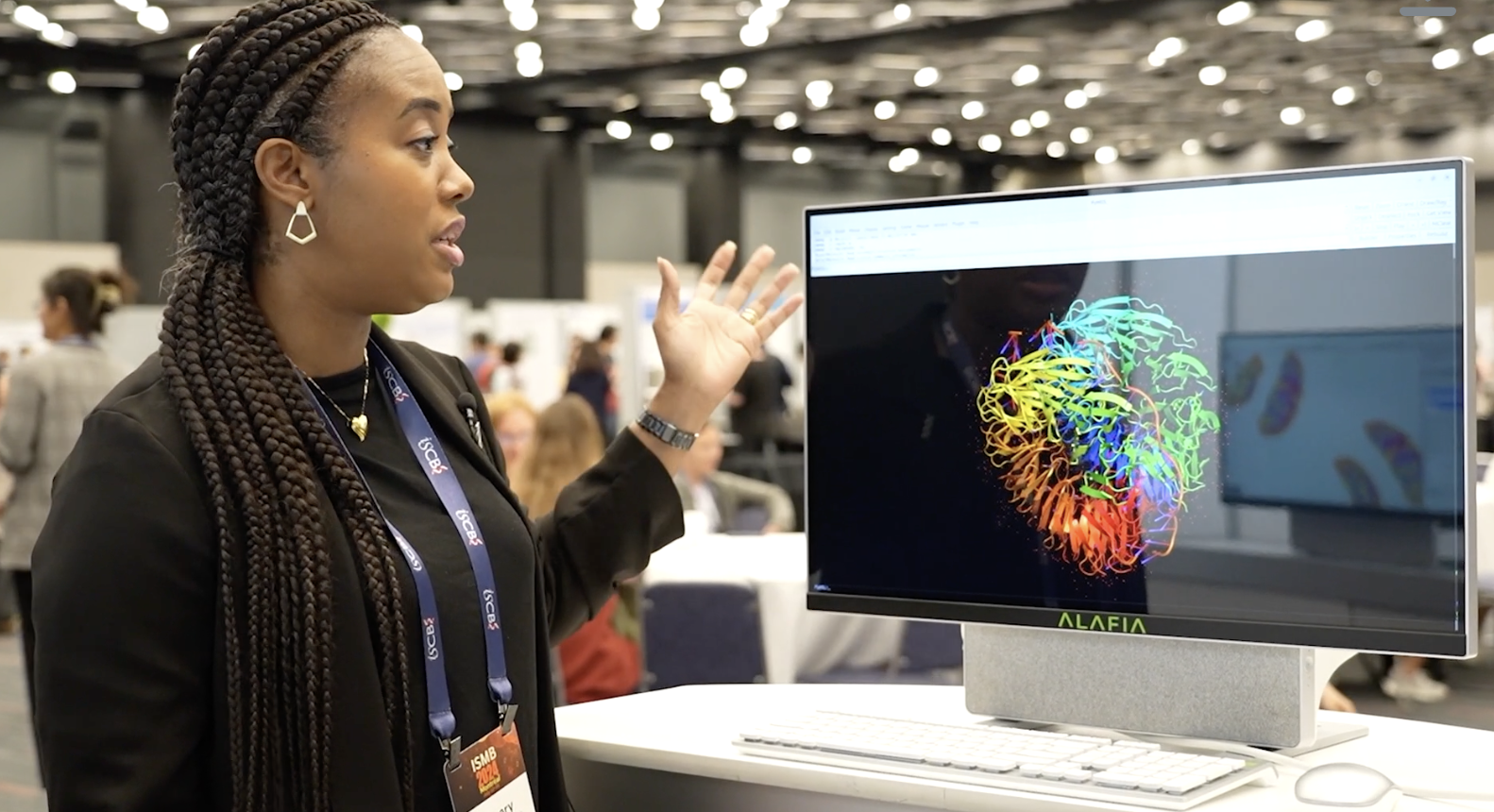
The AIVAS supercomputer
“While imaging centers were eager to develop applications, the real challenge lay in AI. They simply needed better infrastructure, or had underinvested in it. Recognizing this gap, we decided to create a product that would accelerate the development and deployment of AI applications in healthcare. With this solution, once the applications are ready, doctors, pathologists, neurologists, and researchers can run them in real time, locally, on our secure and encrypted systems,” Buscaron explains.
Enhancing workflows with high-performance computational power
The system boasts high-performance hardware, including 128 CPU cores and a staggering 28,416 NVIDIA CUDA GPU cores, paired with 92 GB of high-bandwidth GPU memory. Despite its impressive computational power, it operates efficiently, consuming only 700 watts of energy. In addition, it supports a robust software ecosystem, offering access to more than 1,000 validated software packages that cover a wide array of tasks, from genomic alignment to imaging analysis, ensuring it can handle diverse and demanding workloads.
Early adopters, including Stanford Medical School’s Department of Neurology, report significant time savings. For example, imaging tasks that previously took up to 30 minutes are now completed in under 30 seconds, allowing researchers to achieve results faster and more efficiently, Buscaron adds.
“We began working in pathology because some pathologists had a benchmark for their work. When they ran their post-light image segmentation and cell annotation tests, they were able to complete them in under 30 seconds. The next best computer they could find would take 30 minutes to an hour, which is not a feasible solution for a technician,” he says.
Moreover, as Buscaron points out, machine learning is undoubtedly becoming a key enabler in medicine, as the human body generates far more data than any computer can process alone today. Whether it’s blood test results, imaging data, genomic analysis, biomarkers, or real-time tracking devices, the sheer volume of data generated in real-time is beyond the capacity of any regular computer to analyze comprehensively.
“This creates a significant opportunity. By harnessing that data—much of which holds valuable insights—we can provide doctors with deeper information to help them make better, more informed decisions and improve diagnostic accuracy. The future of healthcare lies in our ability to unlock these insights, driving better outcomes for patients and more efficient medical practices,” he concludes.