Insider Brief
- Drug design, which is expensive and time-consuming, is a potential use case for quantum computers.
- A team of Penn State researchers received a $1.2 million NSF grant to study the use of quantum computers and artificial intelligence for drug design and development.
- The researchers expect to partner with companies, such as IBM and Microsoft, for their study.
- Pictured: the interior of an IBM Quantum computing system. Credit: IBM.
PRESS RELEASE — The time it takes medicines to move from discovery to approved use for patients can take decades and cost billions of dollars. Now, a $1.2 million National Science Foundation grant will help a team of Penn State researchers study the use of quantum computer-based artificial intelligence (AI) to see if quantum computers can bring drugs to patients faster and cheaper.
Quantum computing differs from classical computers because quantum devices use quantum bits — or qubits — rather than classical bits. While bits can only be in binary positions of 1 and 0, qubits rely on the quantum mechanical principle of superposition that allow them to be in 1 and 0 positions simultaneously. Qubits can also be entangled, which means their states are intricately correlated. Theoretically, the devices’ computational potential increases exponentially for every qubit the scientists can entangle.
According to Swaroop Ghosh, associate professor of electrical engineering and computer science and the grant’s principal investigator, these strange — but scientifically proven — abilities give quantum computers the possibility of delivering exponentially more processing power to better handle certain complex problems.
Ghosh added that using artificial intelligence (AI) models designed for quantum computers could be ideal for drug discovery, an area limited by current classical computing processing power.
“Quantum AI models are claimed to be more expressive compared to the classical neural networks -— in other words, they have higher capability to approximate a desired functionality compared to the classical AI models of similar scale,” said Ghosh. “Quantum computers bring effective sampling capabilities so they may be able to model useful distributions of drug-like molecules more efficiently than classical computers. Evolution of quantum primitives, such as quantum memory, can offer further speedup for machine learning tasks since the training data can be directly processed in the quantum domain.”
According to Nikolay V. Dokholyan, G. Thomas Passananti Professor, Penn State College of Medicine and co-principal investigator for the project, quantum computers could cut down on costs associated with finding drugs, as well as shorten the time it takes these needed treatments to reach patients.
“Drug discovery is a lengthy process that can span a decade and costs billions of dollars,” said Dokholyan, who is also an associate of Penn State Institute for Computational and Data Science (ICDS). “Currently, the pace of Federal Drug Administration (FDA) approval of new compounds is only about 40 novel compounds per year. Accelerating drug discovery by computationally screening a massive number of compounds promises to significantly reduce the costs and time for finding effective new cures against diseases. Unlike the traditional computational drug screening approaches that target libraries of billions of compounds, utilizing Quantum Computers along with novel AI-driven algorithms promise to cover a vastly larger chemical space.”
Creating drugs quickly and cheaply means that lives will be saved, according to Ghosh.
“We lose millions of lives every year to diseases like cancer, for example, and the recent pandemic also indicated that we are ill-prepared to find effective drugs quickly,” said Ghosh.
The researchers are particularly interested in leveraging quantum AI to design drug treatments that could inhibit Ras family of proteins, which would be important for cancer treatments and cures.
Quantum AI-designed therapies will not happen overnight, the researchers warn. Several challenges face the use of the technology, Ghosh said. Currently, quantum computers that are available currently are termed “NISQ,” or “noisy intermediate-stage quantum computers.” NISQ devices tend to offer a limited number of qubits to process computations and, because of their extreme sensitivity, require considerable error correction to make up for mistakes introduced by environmental noise, such as heat or electromagnetism.
“Quantum AI suffers from issues that are more fundamental in nature, such as unavailability of training data in the quantum form, all the way to resource-related issues such as limited number of qubits and long wait queue for access to real hardware,” he added.
However, quantum AI may be more expressive, meaning the technology can model a target probability distribution even with a limited number of qubits and parameters to explore possible solutions.
Despite the challenges, the team is excited to take these first steps toward exploring the combined power of artificial intelligence and quantum computing.
“Quantum AI’s true potential in solving real-world problems remains unexplored,” said Ghosh. “This project will fill this void by exploring various quantum AI models that will employ noisy quantum computers.”
The team plans to use quantum computers and quantum computer software tools from companies, such as IBM and Microsoft.
The work was initially supported through seed grants from ICDS and the Huck Institutes.
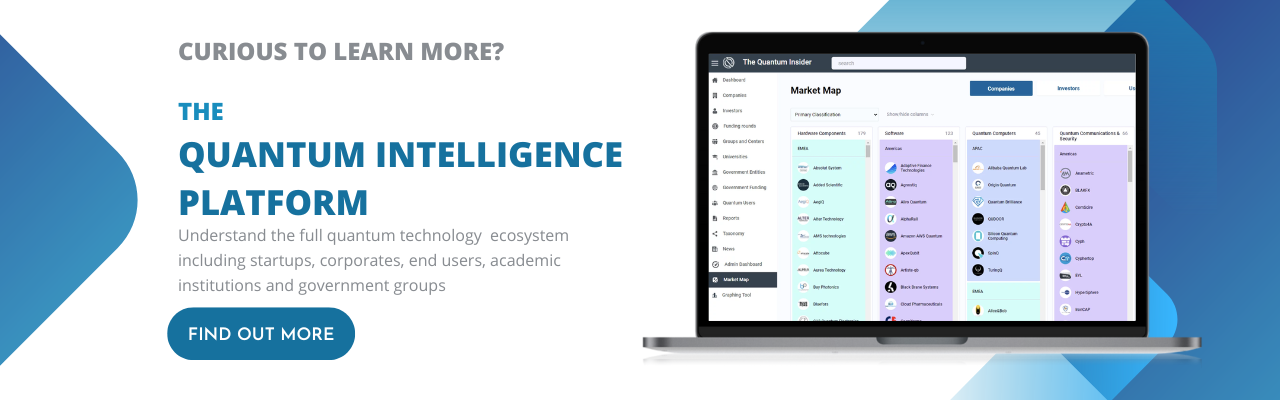
