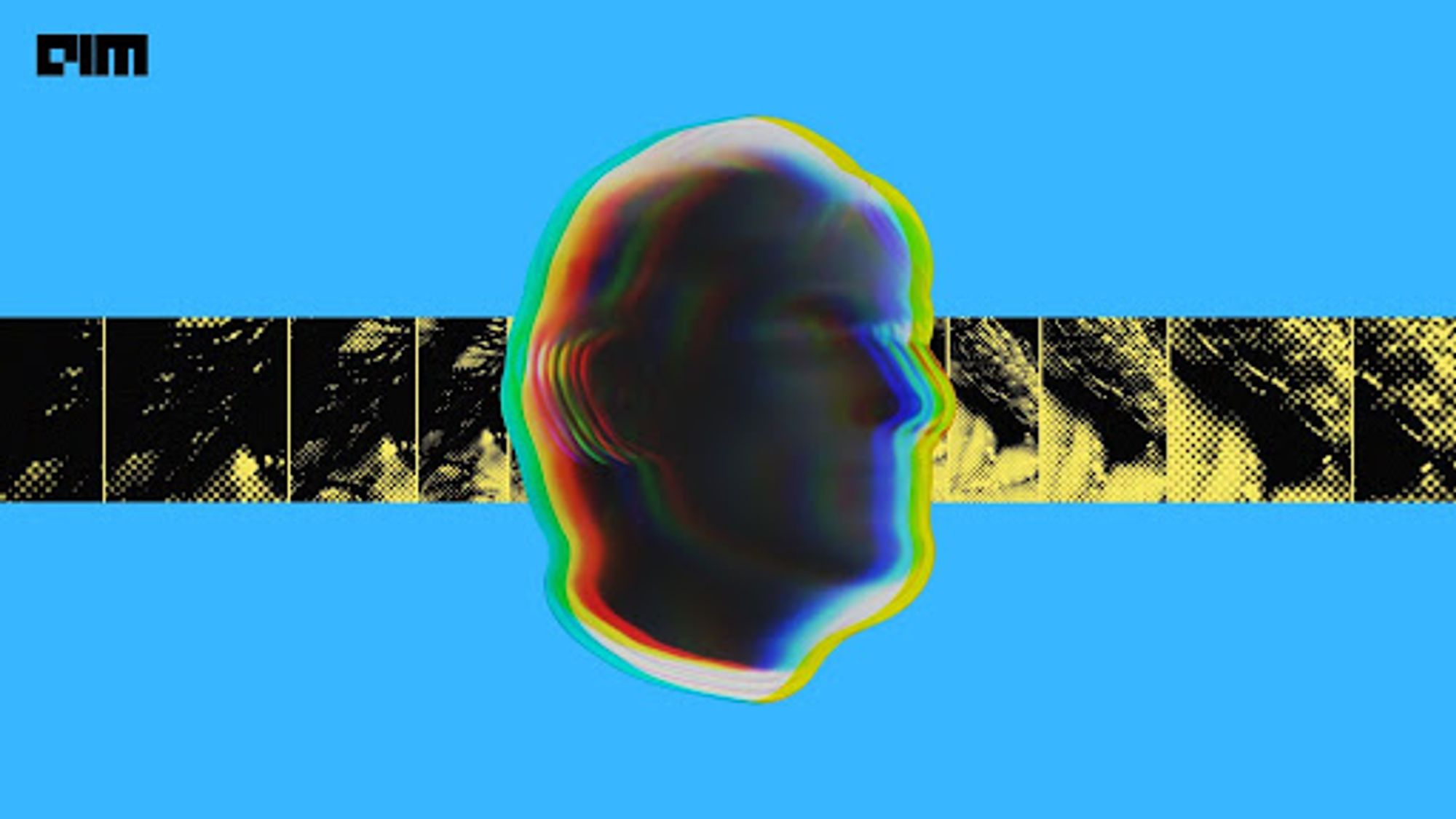
Last year, when Meta AI came up with GSLM or Generative Spoken Language Model, it was the only audio-based language model that was textless. GSLM was able to use raw audio signals directly without any labels. Last week, Meta AI announced three important improvements to GSLM that could help NLP models capture expressions in speech like laughter, yawns or pauses to make communication more nuanced and richer. AI systems prior to this were unable to capture this data because traditional language models like GPT-3, BERT and RoBERTa worked with written text.
Meta AI made a note of three important touchpoints for GSLM in their announcement that included:
- A now open-sourced textless Pytorch-based library on GitHub for speech developers to build on top of GSLM’s building blocks which comprise of a speech encoder that converts speech input to discrete units, a language model that is based on units and a decoder which converts these units back into speech.
- More importantly, GSLM is now also able to model emotional vocalisations that are nonverbal. Whether a sentence is said with anger or happiness depends upon the different vocabulary used, cries, grunts and other nonverbal cues like pauses or tonal quality. These signals help convey the mood of the speaker as irritable, bored or moody.
- GSLM will now be able to model more human-like conversation between two AI with occasional pauses and overlaps. This data can also consequently help voice assistants understand speech that contains overlaps and interruptions while also being able to distinguish between positive and negative feedback.
Method
Text-based NLP doesn’t have the ability to capture context and represents these layers of the text insufficiently. It is also a strenuous task to annotate all emotional expressions in a text. This is why researchers at Meta AI tried to look at the problem from a different perspective. The team modelled all the layers from raw audio at the same time and found that they could achieve realistic audio rendering as the outcome. The study and its findings were put together in a paper titled, ‘Textless Speech Emotion Conversion using Discrete & Decomposed Representations’, which was published in November last year.
Once the input signal is encoded, a sequence to sequence (S2S) model is employed to translate between the sequences that correspond to a different emotion each. Then the duration is predicted, and it arrives at F0 before the signals are fed into a vocoder (G). The pink coloured blocks in the illustration represent models while the green coloured blocks indicate representations.
Speech emotion conversion
The model used a decomposed representation of the speech approach to synthesise speech in the target emotion. While processing the input speech, it considers four parts: phonetic content, prosodic features, which include the pitch, speaking rate as well as the duration, the identity of the speaker and emotion label.
The study suggested a technique that worked in this manner:
- First, extract the emotion from the raw audio waveform using a self-supervised learning model.
- Translate the non-verbal expressions while keeping the lexical content (Example: When amused speech is converted into sleepy, the model removes the laughter and replaces it with yawning).
- Then the prosodic features of the target emotion are predicted after looking at the translated speech.
- Synthesising the speech using the translated speech, prosodic features, target speaker identity and target speaker label.
Conclusion
The study found a new mapping function to translate between discrete speech units from one emotion to another. The results of the study concluded that the method used showed results that outperformed the baselines by a wide margin. The system was eventually able to model expressive non-verbal communication successfully and come up with expressive speech samples of high quality.
The research contributes to speech emotion conversion and improvement while building better GSLMs. The team intends to continue their work and build an end-to-end system to jointly model content units together with prosodic features and use non-parallel datasets.
The model used content, nonverbal cues and timing in a holistic and natural way. It used two identical transformers, one for each stream of speech units, that were automatically derived as in GSLM. Once the model is prompted by 10 seconds of actual conversation, it goes on with its own version. The model is able to naturally have turn durations, gaps of distribution and overlapping speech. All of these can signal agreement, disagreement or even more enthusiasm about the topic or the willingness to take over the conversation.
The wider use of textless NLP will lessen the need for text labels that use way more resources for dubbing or speech-to-speech translation. Besides, language models normally also miss out on this valuable data. If fully explored, textless NLP can be an improvement over the usual systems like Natural Language Processing and Automatic Speech Recognition.