Researchers successfully trained a machine learning algorithm to predict which cancer patients would respond to treatment. Oftentimes, it can be difficult for doctors to know which patients with melanoma would respond well to treatment options.
The study was published in the journal Science Advances.
The open-source deep-learning (DL) program is called DeepTCR. The software tool utilizes algorithms to analyze T-cell receptors (TCRs) sequencing data. The program also taught researchers about the biological methods underlying patients’ responses to immunotherapy.
The immune system and responding to immunotherapy
The study was groundbreaking, not only for the deep-learning predictability, but also because it allowed researchers to see what the model learned about the immune system. “DeepTCR’s predictive power is exciting,” said Dr. John-William Sidhom, first author of the study, "but what I found more fascinating is that we were able to view what the model learned about the immune system’s response to immunotherapy."
He also mentioned the great potential for creating future medications with the information. “We can now exploit that information to develop more robust models, and possibly better treatment approaches, for many diseases, even those outside of oncology.”
Developing DeepTCR
DeepTCR was developed by Dr. Sidhom while he was an M.D./Ph.D student at the Johns Hopkins University School of Medicine. The program uses artificial intelligence to notice patterns in substantial amounts of data. Specifically in the case of this programming, the data is the amino acid sequences of proteins called T-cell receptors, also called TCRs. These receptors sit on the outer surface of the immune system’s T-cells. While there, the TCRs wait to be engaged by a protein from an enemy, such as bacteria, viruses, or cancer.
The T-cell’s surface is covered with numerous TCRs, but they are all identical and all can be attacked by the same enemy. Different T-cells roam the body to wait for enemies. When a TCR is activated, its T-cell releases molecules to destroy and kill the enemy, then it clones itself to strengthen its structure for future attacks and bolster the response.
Some tumor cells create ways to block the T-cells’ response, even when the TCRs are activated. Current immunotherapy drugs called checkpoint inhibitors contain proteins that thwart off tumors, causing T-cells to react to the cancer.
The details of the study
In the study, Dr. Sidhom used materials collected during prior clinical trials that tested the effectiveness of an immunotherapy drug, known as nivolumab. He compared the results to a combination of two drug treatments, consisting of nivolumab and ipilimumab. There were 43 patients who were tested in the study. Each individual had inoperable melanoma.
Biopsies of tumors containing T-cells were taken before and during treatment. In the preliminary study, there were no significant differences in patients who took either one or two drug treatments.
Dr. Sidhom used genetic sequencing to discover the collective TCR repertoire surrounding each tumor by determining the type and number of TCRs in the biopsies.
TCR repertoire.Science Advances/Nature Communications
He then fed the information to the DeepTCR program and told it which datasets belonged to responders of the treatment, in comparison to those who were nonresponsive. Once the input was complete, the algorithm began to look for patterns, initiating predictability.
Questions asked by the researchers
The researchers asked the AI if there were differences before treatment between the TCRs of immunotherapy in responders verses nonresponders. The differences identified by the algorithm were as predictive of patient responses as known biomarkers, or molecular characteristics of tumors used to guide therapy. The researchers wanted to be sure of the results and believe that the algorithm would need to be tested on a larger patient population to assure accuracy and guide therapy.
“These DeepTCR findings define a new dimension for predicting a tumor’s response to immune checkpoint blockade by applying a novel artificial intelligence strategy to deconvolute the vast array of receptors expressed by tumor-infiltrating T cells, the key immune components responsible for direct killing of tumor cells,” said Dr. Drew Pardoll, professor of oncology and director of the Bloomberg~Kimmel Institute for Cancer Immunotherapy.
Responders and nonresponders in the study
Dr. Sidhom wanted to find out the differences between responders and nonresponders. He used data from a past study that linked TCRs to enemy proteins that activated them. The datasets included thousands of TCRs, with each one responding to a different protein from a variety of invaders, which included tumors, the yellow fever virus and the flu virus.
The outcome was contrary to what Sidhom thought would happen. The patients who responded to immunotherapy were those with a higher number of virus-specific T-cells within the tumors. Nonresponders had more tumor-specific T-cells. Both responders and nonresponders had almost the same number of tumor-specific T-cells before and during therapy, he mentioned. “The identity of those T cells remained the same in the responders, but in the nonresponders, there was a different variety of T cells before and during therapy.”
This led to the hypothesis that nonrepsonders had a higher number of ineffective tumor-specific T-cells from the beginning. When the patients who were nonresponders received immunotherapy, their immune systems sent out a new bunch of T-cells, in an attempt to find an effective one. On the other end, responders had effective T-cells from the start, but the anti-tumor activity was blocked by the tumors. When immunotherapy started, it released the blockade and allowed the T-cells to function accordingly.
Future outcomes and predictability
“The application of the deep-learning framework in DeepTCR to characterize the TCR repertoire of T cells allows for improved stratification of patient outcomes along with model explainability in terms of identifying the predictive features,” said Dr. Alexander Baras, associate professor of pathology at the Johns Hopkins University School of Medicine and director of precision medicine informatics at the Johns Hopkins Kimmel Cancer Center.
The study reveals the significant data that artificial intelligence can provide to cancer biology, along with many other medical fields.
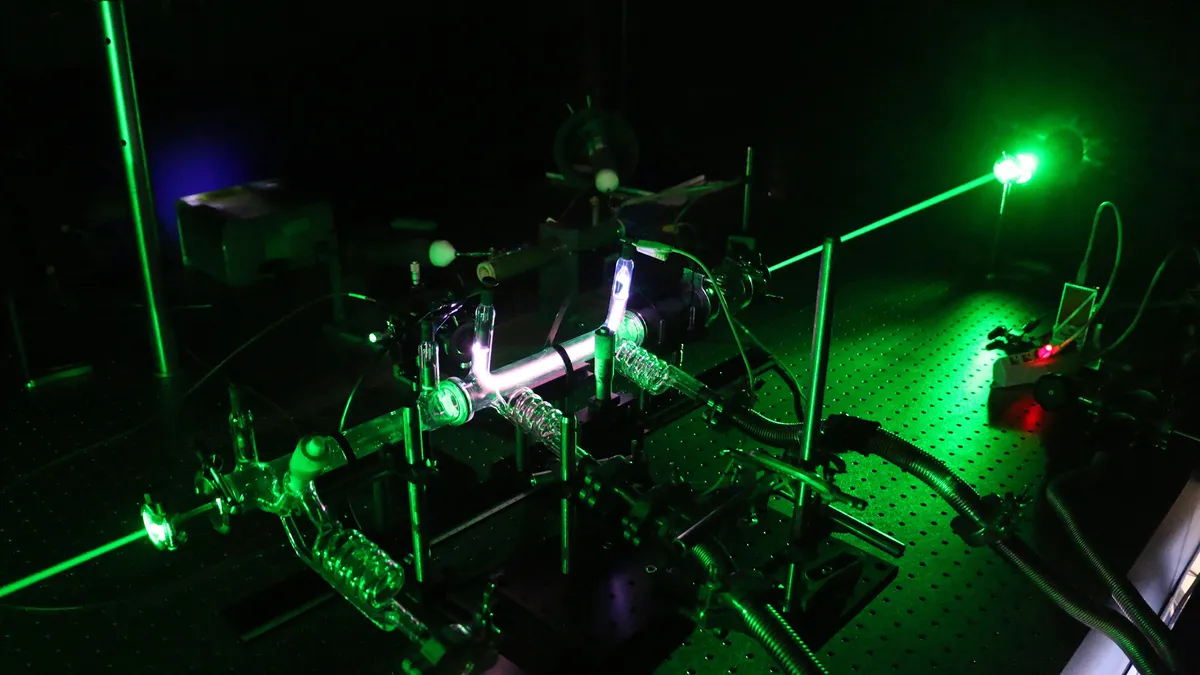
An international team of researchers have introduced a plasma-based method that could convert carbon dioxide into oxygen and produce fuels on Mars.